Ferdinando Fioretto
Assistant Professor of Computer Science, University of Virginia
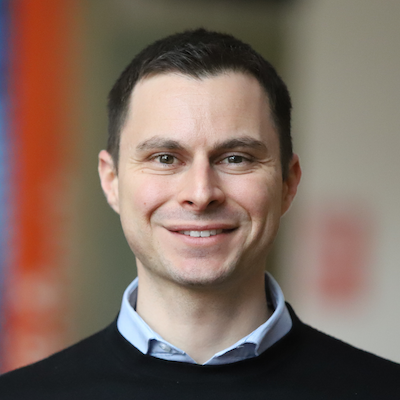
307 Rice Hall
85 Engineer's Way
Charlottesville, VA 22904
I am an assistant professor of Computer Science at the University of Virginia.
I lead the Responsible AI for Science and Engineering (RAISE) group where we make advances in artificial intelligence with focus on two key themes:
AI for Science and Engineering: We develop the foundations to blend deep learning and constrained optimization for complex scientific and engineering problems.
Trustworthy & Responsible AI: We analyze the equity of AI systems in support of decision-making and learning tasks, focusing especially on privacy and fairness.
I am a recipient of the 2022 AWS Amazon Research Award, the 2022 NSF CAREER award, the 2022 Google Research Scholar Award, the 2022 Caspar Bowden PET award, the 2021 ISSNAF Mario Gerla Young Investigator Award, the 2021 ACP Early Career Researcher Award, the 2017 AI*AI Best AI dissertation award, and several best paper awards. My group is generously supported by various projects from the National Science Foundation, Google, Amazon, and the University of Virginia.
Please follow this link for my short Bio.
Opportunities for Undergaduates and Graduate Students
I regularly accept undergaduates and graduates interns who are willing to work on Responsible AI or Differentiable Optimization topics. If you are interested in working with us, send me your CV and interests and make sure you check our latest work!
Selected Talks
Google TechTalks (May 24) | BuzzRobot (Apr 24) | Toc 4 Fairness Seminar (Nov 23) | ACP Summer School 2023 (Aug 23) | DTU Summer School 2023 (Jun 23) | FAccT 2022 Tutorial (June 22) | CP 2021 Invited Talk (Nov 21) | ACP Early Career Award (Dec 21) |
news
Jul-24 |
- Our paper Learning Joint Models of Prediction and Optimization has been accepted to ECAI 2024! ![]() |
---|---|
Jun-24 |
- Invited to attended the National Academy of Science and the Royal Society US-UK Scientific Forum: Scinece in the Age of AI.
- Two papers accepted at ICML workshops! ![]() |
May-24 |
- I am co-organizing the first Summer School in AI and OR (AISCORE).
- I gave a talk on Disparate impacts of compression in Machine Learning at BuzzRobot. - Two papers accepted to ICML 2024! On The Fairness Impacts of Hardware Selection in Machine Learning, and Disparate Impact on Group Accuracy of Linearization for Private Inference. ![]() |
Apr-24 |
- Our paper End-to-End Learning for Fair Multiobjective Optimization Under Uncertainty has been accepted to UAI 2024! ![]() - I gave a talk on formalizing the principle of data minimization for Machine Learning at the Google Privacy seminar serires. - Our paper Fairness Increases Adversarial Vulnerability has been accepted to IJCAI 2024! ![]() - Our paper Learning Fair Ranking Policies via Differentiable Optimization of Ordered Weighted Averages has been accepted to FAccT 2024! ![]() - New preprint: Metric Learning to Accelerate Convergence of Operator Splitting Methods for Differentiable Parametric Programming ! ![]() |
Mar-24 |
- New preprint: Learning Constrained Optimization with Deep Augmented Lagrangian Methods! ![]() - The fith edition of the Privacy Preserving AI workshop at AAAI-24 has been an exciting event. Check out the website for information about its program! - Check out my piece on Fairness in The conservation. |
Feb-24 |
-
New preprint: End-to-End Learning for Fair Multiobjective Optimization Under Uncertainty! ![]() - New preprint: Projected Generative Diffusion Models for Constraint Satisfaction! ![]() - New preprint: Disparate Impact on Group Accuracy of Linearization for Private Inference! ![]() - New preprint: Learning Fair Ranking Policies via Differentiable Optimization of Ordered Weighted Averages! ![]() - I gave a talk at Amazon Science on the disparate impacts of private machine learning. |
Jan-24 |
-
New preprint: Analyzing and Enhancing the Backward-Pass Convergence of Unrolled Optimization! ![]() |
Dec-23 |
- Paper accepted to AAAI 2024! ![]() - New preprint: On The Fairness Impacts of Hardware Selection in Machine Learning! ![]() - ![]() |
Nov-23 |
-
New preprint on integrating prediction and optimization via proxy learning! ![]() - ![]() - Our paper on disparate impacts arising in energy optimization has been accepted to the NeurIPS 2023 Climate Change AI Workshop! ![]() |
Oct-23 |
-
![]() |
Sep-23 |
-
Our paper on data minimization at inference time has been accepted to NeurIPS 2023! ![]() - I am co-organizing the fith edition of the Privacy Preserving AI workshop at AAAI-24. ![]() - Paper accepted in IEEE PES Innovative Smart Grid Technologies. ![]() |
Aug-23 |
- I gave a talk on Privacy and Fairness at the IJCAI-23 workshop on Deep Learning Methods for Social Media.
- New survey on integrating prediction and optimization in end-to-end differentiable systems! ![]() - I am co-organizing the Algorithimc Fairness through the Lens of Time workshop at NeurIPS to spark discussions on how a long-term perspective can help build more trustworthy algorithms in the era of generative models. - New preprint on the disparate impacts arising in energy optimization. ![]() - ![]() |
Jul-23 |
- I gave a talk about the integration of Machine Learning and Optimization at the 2023 ACP Summer School.
![]() |
Jun-23 |
- Paper accepted to Electric Power Systems Research! ![]() - I gave a talk about Differential Privacy, Foundation and applications in Energy Systems at the DTU PET Summer School. ![]() - I gave a talk about Machine Learning for Optimization Optimization at the IEEE PES University. |
May-23 |
- Two new exciting preprints on privacy and fairness. ![]() - ![]() - Our NSF-ENG EPCN proposal Physics Informed Real-time Optimal Power Flow has been funded! We’ll integrate machine learning and physics to optimize power flow, integrate systems dynamics, and increase grid reliability. We’ll work on scalable and robust ML for energy solutions. Thank you, NSF! |
Apr-23 |
- ![]() - Four papers accepted to IJCAI 2023! ![]() |
Mar-23 |
- Our NSF RI CORE proposal End-to-end Learning of Fair and Explainable Schedules for Court Systems. has been funded! We’ll develop differentiable optimization tools for equitable & explainable scheduling and work on changing the pretrial scheduling process to reduce nonappearance and promote fairness in the American Court system! Thank you, NSF!
- I am co-editing a new book ![]() |
Feb-23 | - I have co-organizing the fourth workshop on Privacy Preserving Artificial Intelligence (PPAI) at AAAI-23. |
Jan-23 |
- Four new exciting preprints on topics including differentiable optimization, data leakage in ML models, differential privacy in language models, and differentially private data disclosure methods. ![]() ![]() - Paper accepted to IEEE PES 2023! ![]() - I gave a talk about Differential Privacy and Fairness in Energy Systems at the Grid Science winter school. - I will be serving as an area chair for FAccT-23 and ECAI-23. - I will be serving as demo track co-chair for IJCAI-23 and scholarship co-chair for AAMAS-23. - Paper accepted to AAMAS 2023! ![]() |